Glossary of Artificial Intelligence (AI) terms
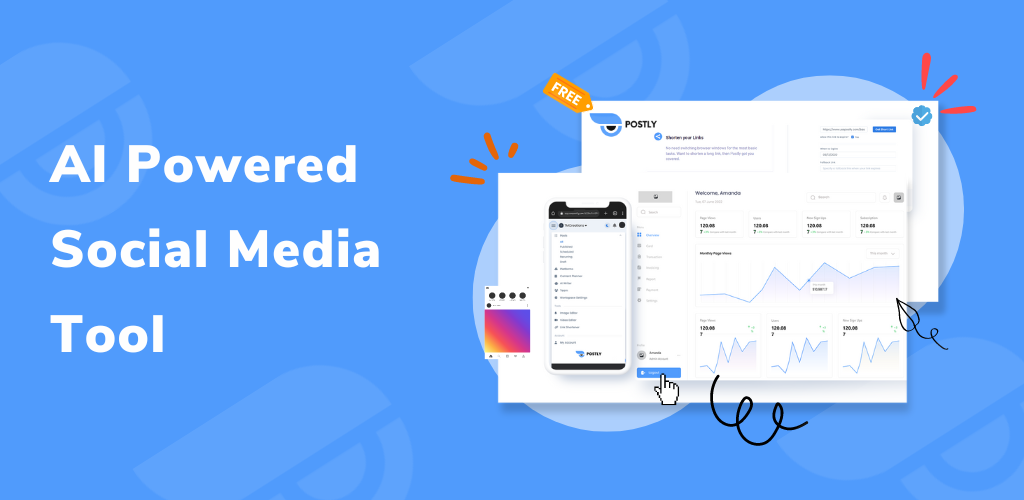
AI Glossary
- AI (Artificial Intelligence): The simulation of human intelligence processes by machines, typically computer systems, to perform tasks that would normally require human intelligence.
- Machine Learning (ML): A subset of AI that involves training algorithms to learn from data and make predictions or decisions without being explicitly programmed.
- Deep Learning: A subfield of machine learning that uses artificial neural networks with multiple layers to model and solve complex tasks.
- Neural Network: A computational model inspired by the human brain, consisting of interconnected nodes (neurons) used for various AI tasks.
- Supervised Learning: A machine learning technique where the algorithm is trained on labeled data, learning to make predictions based on input-output pairs.
- Unsupervised Learning: A machine learning technique where the algorithm learns patterns and structures within data without labeled outputs.
- Reinforcement Learning: A machine learning paradigm where an agent learns to make decisions through trial and error, receiving rewards or penalties.
- Natural Language Processing (NLP): A subfield of AI that focuses on the interaction between computers and human language, enabling machines to understand, interpret, and generate text or speech.
- Computer Vision: A field of AI that allows machines to interpret and understand visual information from the world, such as images and videos.
- Artificial General Intelligence (AGI): A form of AI that has the ability to understand, learn, and apply knowledge in a way that is indistinguishable from human intelligence.
- Artificial Narrow Intelligence (ANI): AI systems that are specialized and excel at a single specific task but lack general intelligence.
- Algorithm: A set of step-by-step instructions for solving a specific problem or completing a task in AI and computer science.
- Feature Engineering: The process of selecting and transforming relevant data features for use in machine learning models.
- Data Preprocessing: Cleaning, transforming, and organizing data to make it suitable for machine learning algorithms.
- Overfitting: A common issue in machine learning where a model performs well on training data but poorly on unseen data due to excessive complexity.
- Underfitting: The opposite of overfitting, where a model is too simple to capture the underlying patterns in data.
- Bias (in AI): Systematic errors in predictions or decisions made by AI systems, often due to biased training data or algorithms.
- Variance (in AI): The sensitivity of a machine learning model to variations in the training data, which can lead to overfitting.
- Supervised Learning Algorithm: Algorithms such as Linear Regression, Decision Trees, and Neural Networks that are used in supervised learning tasks.
- Clustering: An unsupervised learning technique that groups data points into clusters based on similarity.
- Classification: A supervised learning task where the goal is to assign labels or categories to input data.
- Regression: A supervised learning task that aims to predict a continuous numerical output.
- Convolutional Neural Network (CNN): A type of neural network designed for processing grid-like data, such as images.
- Recurrent Neural Network (RNN): A neural network architecture suitable for sequence data, where information is passed from one step to the next.
- Transfer Learning: The practice of using a pre-trained model as a starting point for a new machine learning task.
- Autoencoder: A neural network architecture used for dimensionality reduction and feature learning.
- Gaussian Distribution: A probability distribution often used in statistics and machine learning to model data.
- Logistic Regression: A regression model used for binary classification tasks.
- Natural Language Understanding (NLU): A subset of NLP focused on enabling AI to comprehend and interpret human language.
- Natural Language Generation (NLG): The AI-driven process of generating human-like text or speech.
- Speech Recognition: AI technology that converts spoken language into written text.
- Chatbot: An AI application designed to engage in text or voice-based conversations with users.
- Recommendation System: AI software that suggests products, content, or services based on user preferences and behavior.
- Big Data: Extremely large and complex data sets that require specialized processing techniques, often used in AI and machine learning.
- Semi-supervised Learning: A combination of supervised and unsupervised learning, where a model is trained on both labeled and unlabeled data.
- Ensemble Learning: The practice of combining multiple machine learning models to improve predictive performance.
- Decision Tree: A graphical representation of decisions and their consequences, often used for classification and regression tasks.
- Random Forest: An ensemble learning method that combines multiple decision trees to improve accuracy and reduce overfitting.
- Gradient Descent: An optimization algorithm used to train machine learning models by adjusting model parameters.
- Loss Function: A mathematical function that measures the difference between predicted and actual values, used in model training.
- Reinforcement Learning Agent: The AI entity that interacts with an environment and learns to maximize a cumulative reward.
- Markov Decision Process (MDP): A mathematical framework used in reinforcement learning to model decision-making problems.
- Q-Learning: A reinforcement learning algorithm used to learn optimal action-selection policies in an MDP.
- Deep Reinforcement Learning: The combination of deep learning and reinforcement learning, often used in game-playing AI.
- Artificial Neural Network (ANN): A computational model inspired by biological neural networks, used in machine learning and AI.
- Perceptron: The simplest form of a neural network, used for binary classification.
- Backpropagation: The process of updating neural network weights to minimize errors during training.
- Long Short-Term Memory (LSTM): A type of recurrent neural network architecture designed to capture long-term dependencies in sequential data.
- Gated Recurrent Unit (GRU): Another type of recurrent neural network that simplifies the architecture compared to LSTM.
- Word Embedding: A technique to represent words as numerical vectors in NLP, e.g., Word2Vec and GloVe.
- Tokenization: The process of breaking down text into smaller units, such as words or sentences, for analysis.
- Bag of Words (BoW): A simple NLP model that represents text as a collection of words, ignoring word order.
- Named Entity Recognition (NER): The task of identifying and classifying named entities in text, such as names of people, places, and organizations.
- Sentiment Analysis: A form of NLP that determines the sentiment or emotion expressed in text, often used in social media monitoring.
- Part-of-Speech (POS) Tagging: Identifying the grammatical category of words in a text, e.g., nouns, verbs, adjectives.
- Chatbot Framework: Development frameworks like Dialogflow and Rasa for building chatbots and conversational AI applications.
- Word2Vec: An algorithm for generating word embeddings from large text corpora.
- GloVe (Global Vectors for Word Representation): A pre-trained word embedding model.
- Recurrent Neural Network (RNN): A type of neural network that processes sequential data with loops or cycles.
- One-Hot Encoding: A technique used to convert categorical data into numerical format.
- Support Vector Machine (SVM): A supervised machine learning algorithm used for classification and regression.
- Principal Component Analysis (PCA): A dimensionality reduction technique used to simplify data while retaining important features.
- K-Means Clustering: A popular unsupervised learning algorithm for grouping data points into clusters.
- K-Nearest Neighbors (K-NN): A simple classification algorithm that assigns labels based on the majority class of its neighbors.
- Recurrent Neural Network (RNN): A neural network architecture suitable for sequence data, where information is passed from one step to the next.
- Generative Adversarial Network (GAN): A neural network architecture involving two networks, a generator and a discriminator, used for generating synthetic data.
- Fuzzy Logic: A mathematical framework for dealing with uncertainty in data, often used in AI and control systems.
- Turing Test: A measure of a machine's ability to exhibit human-like intelligence, introduced by Alan Turing.
- Markov Chain: A stochastic model that describes a sequence of events where the probability of each event depends only on the previous event.
- Recommender System Algorithm: Algorithms like Collaborative Filtering and Content-Based Filtering used in recommendation systems.
- Natural Language Processing API: Application Programming Interfaces for accessing NLP tools and services, such as Google Cloud NLP.
- Sentiment Analysis Tool: Software that analyzes sentiment in text data, often used in social media monitoring and customer feedback analysis.
- Speech-to-Text: AI technology that converts spoken language into written text, also known as Automatic Speech Recognition (ASR).
- Text-to-Speech: AI technology that converts written text into spoken language.
- Data Mining: The process of discovering patterns and information from large datasets.
- Data Warehouse: A repository for storing and managing large volumes of structured data, often used for business intelligence and data analysis.
- Cloud Computing: A technology that allows the use of remote servers on the internet to store, manage, and process data.
- Edge Computing: Processing data closer to its source, reducing latency and improving efficiency, often used in IoT and AI applications.
- IoT (Internet of Things): A network of physical devices and objects embedded with sensors and connected to the internet for data collection and automation.
- AI Ethics: The study of moral and ethical issues related to the development and use of AI.
- AI Bias Mitigation: Techniques and strategies to reduce bias in AI systems, ensuring fairness and equity.
- Explainable AI (XAI): The capability of AI systems to provide clear, understandable explanations for their decisions and predictions.
- Fairness in AI: Ensuring AI systems do not discriminate against individuals or groups based on factors such as race, gender, or age.
- Machine Ethics: The study of ethical issues arising from the behavior of AI and autonomous systems.
- Data Privacy: Protecting individuals' personal information and ensuring it is used responsibly in AI and data analysis.
- GDPR (General Data Protection Regulation): A European regulation designed to protect the privacy and data of individuals.
- HIPAA (Health Insurance Portability and Accountability Act): A U.S. law that governs the security and privacy of healthcare data.
- Exascale Computing: A level of computing performance that is a billion billion calculations per second (an exaflop).
- Quantum Computing: A type of computing that uses quantum bits (qubits) and has the potential to perform certain calculations much faster than classical computers.
- Heuristic: A problem-solving approach that uses experience-based rules or methods to find solutions, often used in AI search algorithms.
- Natural Language Generation (NLG): The AI-driven process of generating human-like text or speech.
- Speech Synthesis: The artificial production of human speech, also known as Text-to-Speech (TTS).
- Recurrent Neural Network (RNN): A type of neural network architecture suitable for processing sequential data.
- Long Short-Term Memory (LSTM): A type of RNN that is capable of learning and remembering long-term dependencies in data.
- Gated Recurrent Unit (GRU): A simplified version of the LSTM used in RNNs.
- Word Embedding: Techniques such as Word2Vec and GloVe that represent words as numerical vectors in NLP.
- Tokenization: The process of splitting text into smaller units, like words or sentences, for analysis.
- Bag of Words (BoW): A basic NLP model that represents text as a collection of words, ignoring word order.
- Named Entity Recognition (NER): Identifying and categorizing named entities in text, such as names of people, places, and organizations.
- Sentiment Analysis: A form of NLP that determines the sentiment or emotion expressed in text, often used in social media monitoring.
- Part-of-Speech (POS) Tagging: Identifying the grammatical category of words in a text, e.g., nouns, verbs, adjectives.
- Chatbot Framework: Development frameworks like Dialogflow and Rasa for building chatbots and conversational AI applications.
- Word2Vec: An algorithm for generating word embeddings from large text corpora.
- GloVe (Global Vectors for Word Representation): A pre-trained word embedding model.
- Recurrent Neural Network (RNN): A type of neural network architecture suitable for sequence data, where information is passed from one step to the next.
- Generative Adversarial Network (GAN): A neural network architecture involving two networks, a generator and a discriminator, used for generating synthetic data.
- Fuzzy Logic: A mathematical framework for dealing with uncertainty in data, often used in AI and control systems.
- Turing Test: A measure of a machine's ability to exhibit human-like intelligence, introduced by Alan Turing.
- Markov Chain: A stochastic model that describes a sequence of events where the probability of each event depends only on the previous event.
- Recommender System Algorithm: Algorithms like Collaborative Filtering and Content-Based Filtering used in recommendation systems.
- Natural Language Processing API: Application Programming Interfaces for accessing NLP tools and services, such as Google Cloud NLP.
- Sentiment Analysis Tool: Software that analyzes sentiment in text data, often used in social media monitoring and customer feedback analysis.
- Speech-to-Text: AI technology that converts spoken language into written text, also known as Automatic Speech Recognition (ASR).
- Text-to-Speech: AI technology that converts written text into spoken language.
- Data Mining: The process of discovering patterns and information from large datasets.
- Data Warehouse: A repository for storing and managing large
Glossary of Generative AI Terms
- Generative AI: Generative AI refers to a subset of artificial intelligence that focuses on creating new data, such as images, text, or even music, rather than just making predictions or classifications. It often uses generative models.
- Generative Model: A type of AI model designed to generate new data that is similar to a given dataset. Examples include Variational Autoencoders (VAEs) and Generative Adversarial Networks (GANs).
- Variational Autoencoder (VAE): A generative model that combines elements of autoencoders and probabilistic modeling to generate data. VAEs are commonly used for generating diverse and realistic data points.
- Generative Adversarial Network (GAN): A generative model that consists of two neural networks, a generator, and a discriminator, which compete against each other. The generator aims to produce realistic data, while the discriminator attempts to distinguish real from fake data.
- Latent Space: A multi-dimensional space where data is represented in a more abstract form, making it easier for generative models to manipulate and generate new data.
- Conditional Generation: Generating data with certain conditions or constraints. For example, text-to-image generation with a specific textual description.
- Natural Language Generation (NLG): A subfield of generative AI that focuses on generating human-like text or language, often used in chatbots, content generation, and translation services.
- Text Generation: The process of using generative models to create written content, such as articles, stories, or poetry, using AI algorithms like Recurrent Neural Networks (RNNs) or Transformer models.
- Image Generation: Creating images using generative models, including techniques like GANs, where the generator network generates images from random noise.
- Style Transfer: A technique used in generative AI to apply the artistic style of one image to the content of another, creating visually appealing results.
- Autoencoder: A type of neural network used in generative models that compresses data into a lower-dimensional representation (encoder) and then reconstructs it (decoder). Autoencoders can be used for data generation and denoising.
- Sequence-to-Sequence (Seq2Seq): A neural network architecture used for various generative tasks, like machine translation and text summarization, where it takes a sequence of input data and generates a sequence of output data.
- Conditional GAN (cGAN): A variation of GAN where the generator and discriminator are conditioned on specific data or labels, allowing for more controlled data generation.
- Recurrent Neural Network (RNN): A type of neural network architecture often used for sequential data generation, such as text generation, due to its ability to maintain context over time.
- Long Short-Term Memory (LSTM): A specific type of RNN that is capable of learning long-range dependencies in data, making it effective for sequence generation tasks.
- Transformer Model: A deep learning architecture that has been highly successful in natural language processing and is used for various generative tasks, such as text generation and machine translation.
- Attention Mechanism: A component used in many generative models, like Transformers, that allows the model to focus on specific parts of the input data when generating output, improving accuracy and coherence.
- Data Augmentation: A technique used in generative AI to create additional training data by applying transformations or perturbations to the existing data, improving the model's generalization.
- Mode Collapse: A common issue in GANs where the generator produces a limited variety of outputs, failing to capture the full diversity of the training data.
- Top-k Sampling: A method used in text generation to select the top-k most likely words as the next word in a sequence, enhancing diversity and control in the generated text.
- AI Prompt: An AI prompt is a specific instruction or query provided to an artificial intelligence system, like a language model. It serves as a request for the AI to generate a response, complete a task, or provide information based on the input it receives. The quality and clarity of the prompt greatly influence the AI's output. Well-crafted prompts are essential to obtain accurate and relevant responses from AI models.
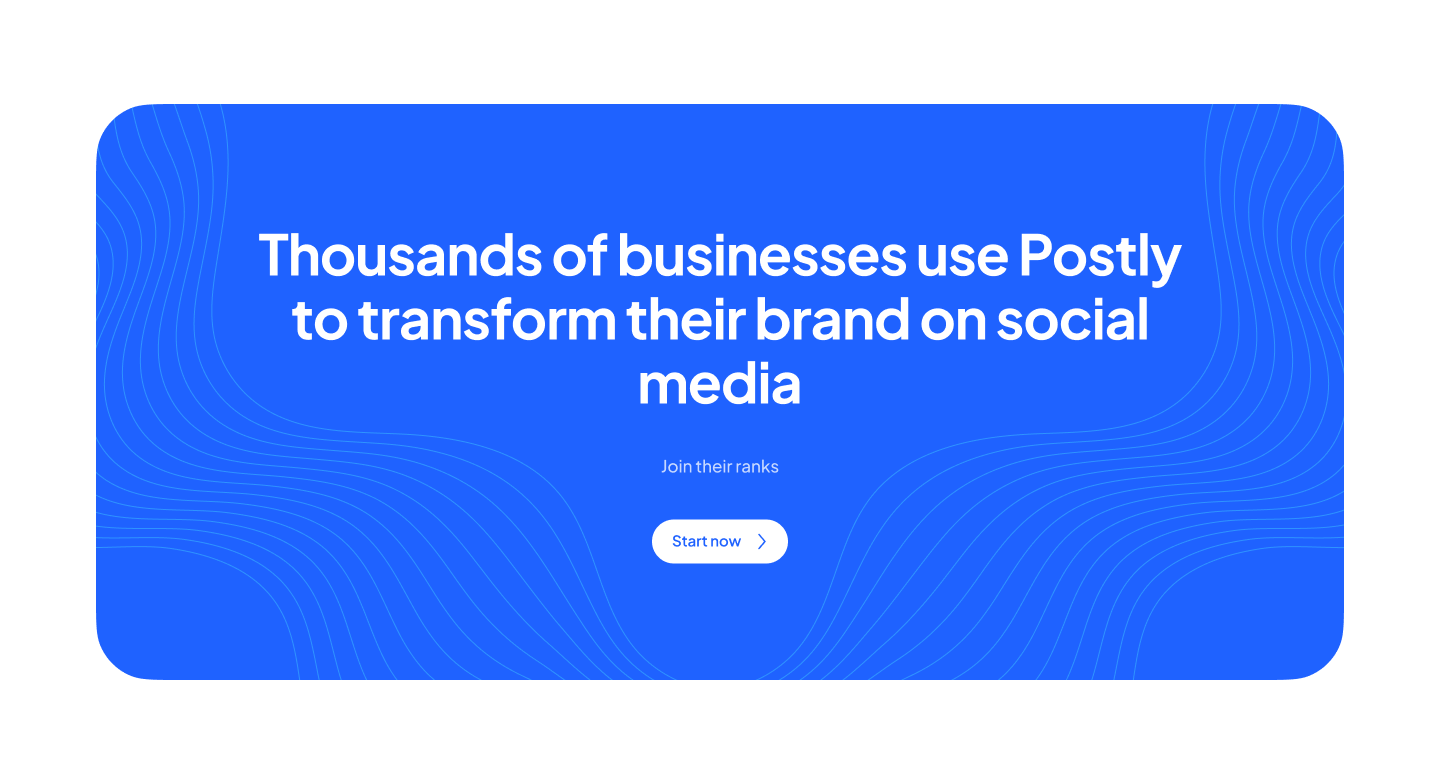